How Artificial Intelligence could affect our everyday lives
From disinformation to DALL-E, what might our futures look like in a world powered by AI?
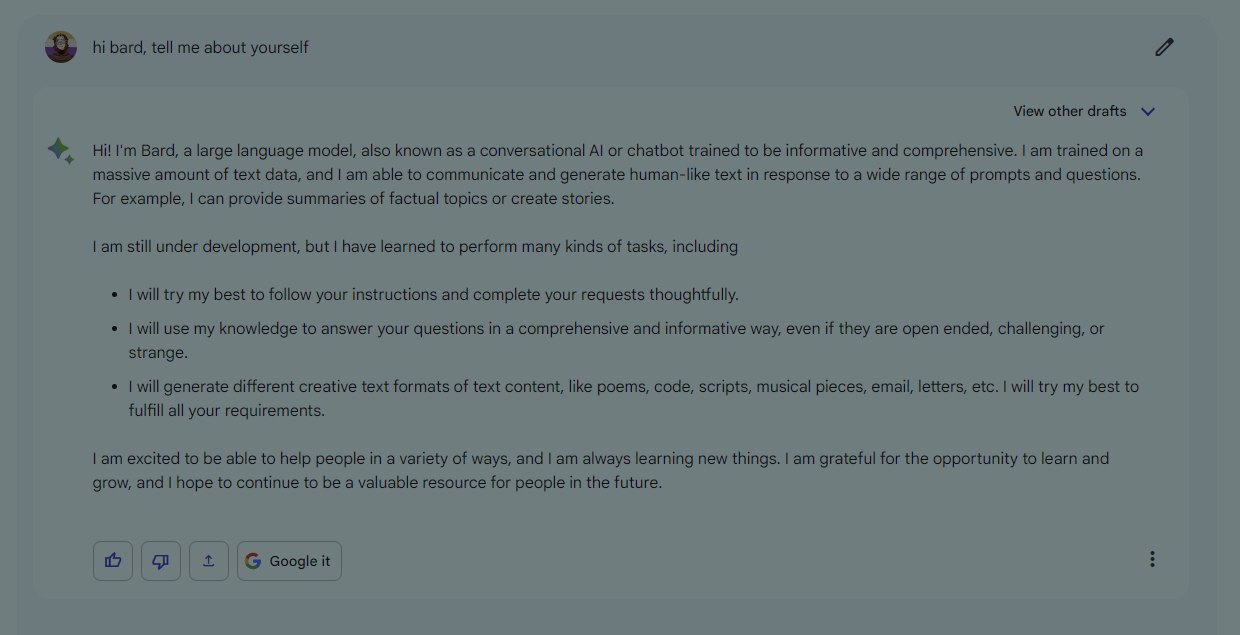
Once considered a thing of the future, Artificial Intelligence is now present in many aspects of our lives. From infamous chatbots like ChatGPT to everyday essentials like Google Search, this technology powers many of the programmes we have come to rely on and enjoy.
To summarise it simply, AI has one goal: replicating natural, human intelligence. It does so by deciding its responses based on information it has been fed- be it websites like Wikipedia, social media platforms, or conversations with the public. This process forms the basis of novel, popular programmes like Google’s Bard and OpenAI's DALL-E, to name a few.
Between the excitement of artificial Drake tracks and award-winning fake photographs, however, lies a more sinister side of AI. A report by Goldman Sachs recently predicted that automation could replace the equivalent of 300 million full-time jobs in the US and Europe, and world organisations have warned against AI’s potential biases and capability to be used to spread misinformation. In this light, young adults are rightfully beginning to worry what their futures might look like in an AI-world.
So, what can we expect as Artificial Intelligence continues to develop?
Computer-generated journalism
You might believe AI tools can’t replace journalists because they lack the critical thinking, creativity, and relationships necessary for good journalism.
However, that introduction was written by Bard, taken from an entire news article the programme generated for me on the relationship between journalism and AI. When asked to explain how it came up with this first sentence, Bard claims it first generated “a list of possible sentences that could be used to complete the thought”, then selected the one it thought was “the most accurate and concise.” In some ways, using the same processes I did to write this article- just in a few seconds, rather than the few days it has taken me.
Some newsrooms have already decided to adopt AI into their general practice, using it to personalise and enhance content. Others only intend to dip their toes in, still wary of the technology’s potential limitations. Those who have chosen to publish articles produced entirely by ChatGPT and its counterparts have received widespread criticism from both journalists and consumers- citing inaccuracies, plagiarism, and the replacement of writing jobs as ethical issues associated with the practice.
Nathan Grayson, a former Washington Post journalist, criticises CNET for artificially generating copy
So, are journalists safe from the mass-GPT takeover? Kind of. At this stage, AI cannot chase down leads or write features, and any stories it produces still need to be checked for accuracy and errors. However, with news outlets like CNET attributing almost 80 articles to AI, copywriters might be finding themselves out of luck in the near future.
Software engineering
"I'm painfully aware of the irony… that a huge amount of my industry is dedicated to sewing the seeds of its own destruction," says Johnny Morrison-Howe, a Junior Developer.
As a traditional coder, he feels like he’s managed to “slip through a closing door”. Many of the more routine tasks he completes on a daily basis are likely to become fully automated as advancements are made. He says that in five years, those with his level of experience will probably be harder-pressed to find employment, predicting AI will cut teams of coders “at least in half”.
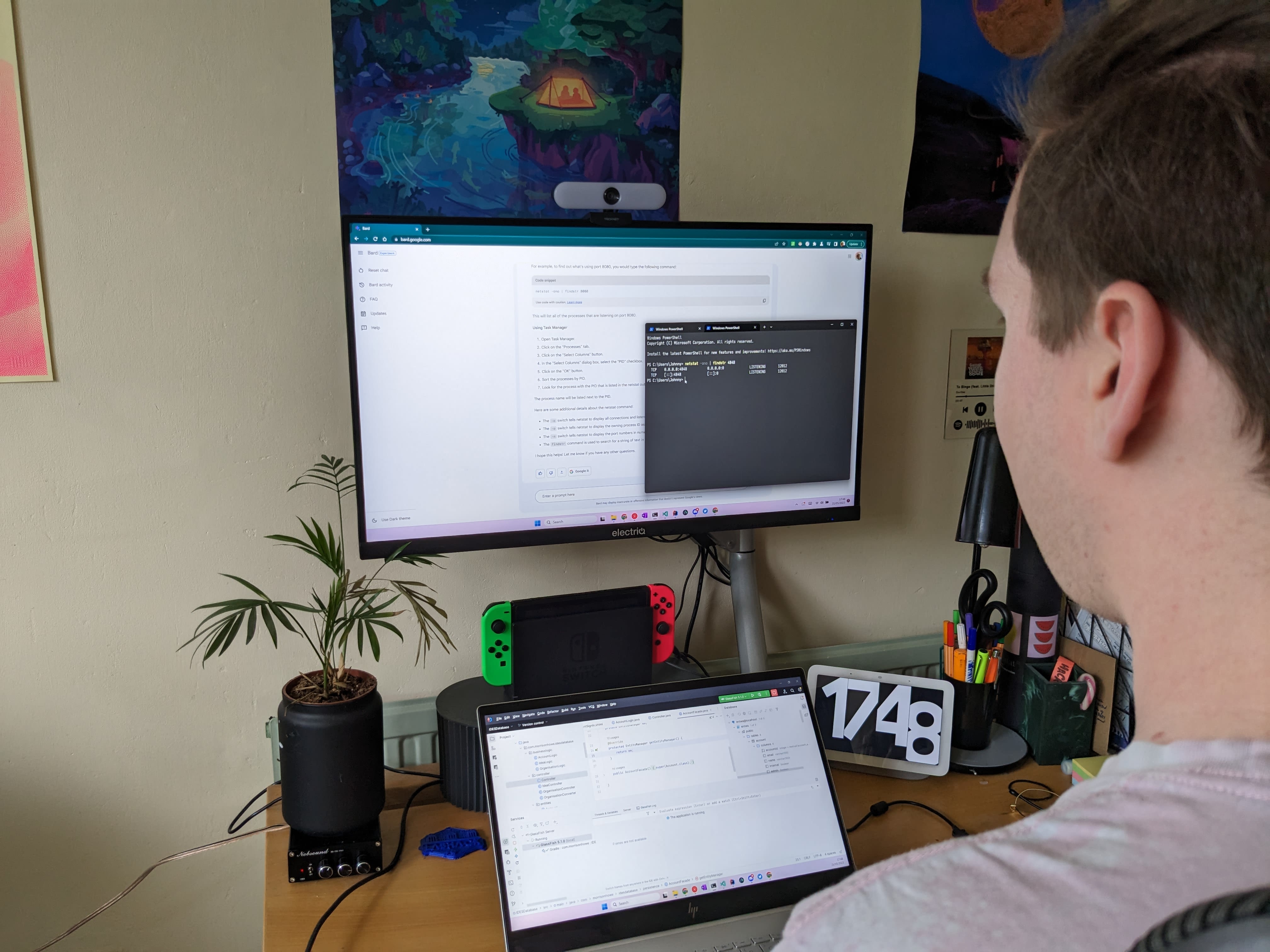
Johnny, a 22-year-old Junior Developer from Portsmouth, in his home office
Johnny, a 22-year-old Junior Developer from Portsmouth, in his home office
That being said, many have acknowledged that there is much more to software engineering than just traditional programming. Johnny, alongside leading businesses in the industry, have acknowledged that the more ‘human’ elements of the job- namely creative problem-solving, design skills, and a broader understanding of how systems interconnect- cannot be automated. Lead AI Scientist Dr. David Ralph adds that whilst software-related tasks (such as code) are more likely to become automated, jobs themselves are not, as they fundamentally involve a high degree of human involvement. He quotes a common expression in the industry: “Programming is thinking, not typing”.
AI tools such as Github’s Copilot also have the potential to greatly aid programmers, from troubleshooting to writing simple lines of code. In this regard, professionals would be freer to undertake larger, more complex projects.
Overall, it seems software engineers are safe for now, and may even benefit from AI in its current form.
AI Art
Artists have undoubtedly been the most impacted by programmes such as DALL-E and Midjourney. Now that it’s possible to turn text prompts into images in seconds, those who rely on commissions as a source of income are at a loss. Many are also seeing their work used to train AI models without their consent.
“AI is actively stealing from people, and there’s just no accountability,” says George Wilson, a digital artist. He describes how on many art-sharing platforms, creators must actively opt-out of having their work scraped by AI programmes. Issues such as this recently led to the #NoToAIArt campaign, which saw artists question the morality and legality of AI-image generators.
As someone who mostly draws fan works, he also believes Artificial Intelligence foregoes the core elements of art, such as creativity and community. He believes commissions are friendlier and more flexible way to receive custom artworks, and says they provide artists with valuable feedback.
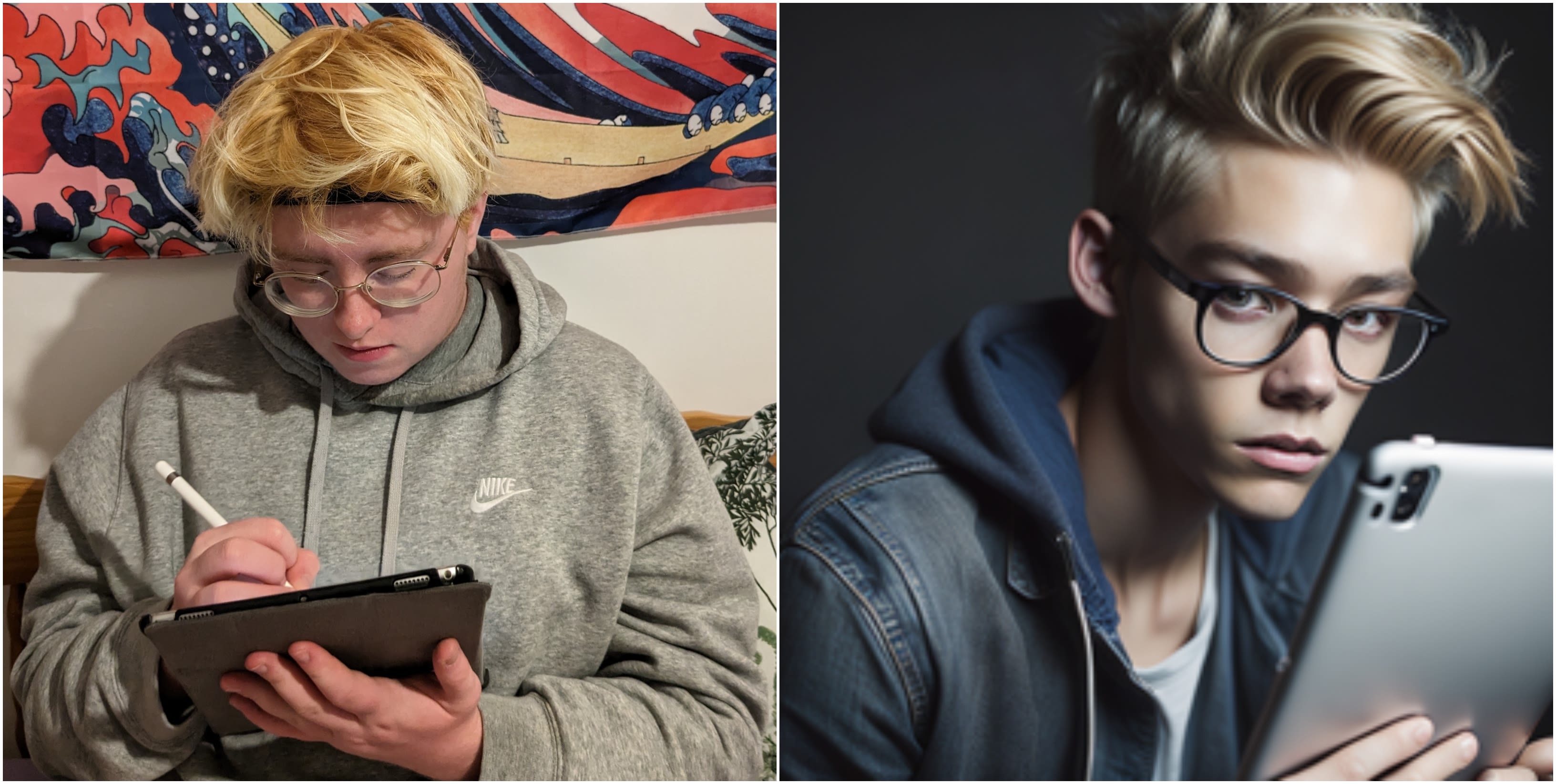
George, a 22-year-old digital artist, and a DALL-E generated portrait of someone with his description
George, a 22-year-old digital artist, and a DALL-E generated portrait of someone with his description
“I don't think that [AI] could ever replicate a true human piece of art,” he concludes.
Whilst many in the creative industry share this sentiment, consumers are increasingly turning to AI as a cost-effective means of receiving custom works. This could spell trouble for artists in the near-future.
Deepfakes, disinformation, and doctored pornography
Although disinformation as an issue long precedes the AI boom, new and accessible tools have made it easier and faster for people to produce convincing falsehoods. Recent viral hoaxes have ranged from a rather innocent artificial image of Pope Francis in a puffer jacket, to a more sinister doctored video of US President Joe Biden. announcing a national draft. The latter is one of many deepfakes- images and videos of false events made using Artificial Intelligence- to have spread online.
A deepfake of U.S. President Joe Biden calling for a national draft. Twitter has added context to let viewers know the video is not real
Deepfakes pose an extensive risk for several reasons. The first of these is their capability to cause political unrest. False depictions of politicians are being shared for political gain, including President Biden giving an anti-transgender speech and Donald Trump being arrested. Given the rate at which disinformation disseminates online, these AI-generated deceptions may potentially interfere with upcoming elections not only in the United States, but also the UK and further afield.
Deepfake pornography has also risen in prominence, with a report from cyber-security company Deeptrace finding 96% of all deepfakes were pornographic in nature in 2019. They found nine deepfake dedicated pornography websites hosting over 13,000 non-consensual doctored videos, and apps specifically used to artificially ‘strip’ photos of women, such as DeepNude. In this regard, AI technologies are being used specifically to control and humiliate women, with potentially disastrous impacts on their mental health.
Dr. Ralph recommends educating the public on how to determine reliable sources of information as an effective solution to disinformation, and campaigners have demanded tougher laws against non-consensual sexual AI content. In the meantime, however, we should prepare for further doctored political and pornographic material to circulate online as the amount of deepfakes online increase exponentially.
For something so prevalent in our lives, many of us still struggle to understand what Artificial Intelligence is. I consulted Lead AI Scientist Dr. David Ralph to break it down.
What is AI?
There are lots of definitions but generally: the goal of Artificial Intelligence is to replicate capabilities of natural intelligence, such as sensing, communicating, and reasoning. We generally divide this into "narrow" AI and "general" AI. Narrow AI is intended to perform a specific task (e.g. classify emails as spam or not spam), whereas general AI would seek to learn new skills and act independently and may theoretically have something resembling consciousness or awareness. All current AI is narrow, even if it may seems like some have very broad capabilities it's just that the tasks they are trained on are very broad (e.g. ChatGPT's task is to predict the most likely next words), they do not seek out new knowledge or have autonomy, and most existing AIs are not actually capable of learning at all once it has finished training. The techniques most widely used in the AI field are generally not considered likely to ever produce general AI and only a small minority of research is concerned with it.
What is Machine Learning?
This is a more specific term than AI and refers to technologies that analyse and draw inferences from patterns in data. Most but not all AI is powered by machine learning. For example, an expert system is a type of algorithm that uses a series of rules to make decisions (like a flowchart), this can replicate the intelligent behaviour of reasoning but does not require machine learning. For example one of those rules might be some numerical threshold (e.g. what level of a particular pollutant is unsafe), this could be a number decided by a human expert (not machine learning) or calculated based on the known outcomes for historical data (machine learning). Popular machine learning techniques include neural networks (which simulate neurons like in biological brains), genetic algorithms (which simulate evolution), decision trees (essentially self-arranging flowcharts), and some other types of statistical algorithms. Deep learning is a subtype of neural networks designed to have more complex architectures that allow them to work with complex raw data like text, images, and sound rather than simple features like measurements. For this reason deep learning is sometimes also called feature-learning, as the model learns how to determine features from raw data itself.
What are Large Language Models?
LLMs are very powerful deep learning models trained on huge amounts of text data (typically most of Wikipedia, public social platforms like Reddit, open access books, journals, and research papers, etc.). Usually these are trained with tasks like predicting a missing word or next word in a sentence, predicting what order sentences should be in, and whether sentences belong together. The idea is that by looking at the patterns in text it's possible to develop a statistical model of language. Because LLMs learn what words are associated with each other, they are also able to learn "world-knowledge", for example a LLM might know the names of all of Shakespeare's plays and associate them with him because it has seen them mentioned together many times and seen how people talk about them. LLMs themselves are not directly useful, so we then fine-tune (retrain) them for the tasks we actually want them to perform by showing them examples of some input (like a text prompt) and an example of a good output (an answer to the prompt), because the model already understands language it is much easier for it to learn the task we want than if it was learning from scratch, which means we need fewer good examples of the task.
How do chatbots like ChatGPT work?
Bard and ChatGPT are fine-tunned models based on LLMs (in this case GPT3), see Q3 for how LLMs work. The additional training applied on top of the LLM consists of finetuning tasks in the form of lots of examples of prompts and good answers, because the LLM already understands language it quickly learns what exactly you are asking it to do, i.e. the format in which it should answer. They then go through a process called reinforcement-learning-from-human-feedback (RLHF) where the model is given prompts and a human judges the output as good or bad (or sometimes multiple answers as better/worse), so the model then learns to produce answers humans would consider good. While the pre-training of LLMs uses a huge amount of mixed quality data, it's important the data used for finetuning and RLHF is good quality and reflects the kinds of things the model needs to do, as the LLM may have a lot of world knowledge you actually don't want the final model to use (e.g. ChatGPT is taught to not answer potentially dangerous questions even if it does know the answer).
Are there any common misconceptions about AI?
Absolutely, here are the ones I hear most often:
- Confusion between general and narrow AI (see answer to Q1), particularly the idea that AI can seek out information or teach/improve itself.
- That an AI learns from your interactions. This is half true, we do often collect responses to train the next generation of models, but models typically don't continue to learn after training, techniques for this are called "active learning" but aren't common in deep learning.
- That researchers don't consider the consequences or ethics of AI. There are entire fields of research, "AI Safety" and "AI alignment", which are concerned with this. Unfortunately commercial and political interests rarely give it sufficient attention, but it absolutely does exist and is taken very seriously by researchers.
- That AI models do not produce original content and/or only remix their training data. This is most often said about generative AI like Stable Diffusion or ChatGPT, but is demonstrably false as the training data for these models is usually more than 1000x or 10000x the size of the model. For images lossless compression can at best achieve around 5x, and lossy compression around 20x before the image is severely distorted. When the training data contains the same or very similar examples many times then the model may partially memorise it, but this is very rare, e.g. I expect Stable Diffusion can reproduce the Mona Lisa because the training data will include many thousands of slightly different photos of it. We call this kind of memorisation "overfitting" and models are trained to avoid this because it's detrimental to the purpose of the model, which is to generate original content.
Some people believe AI is "moving too fast" - as someone who works with it, do you agree?
This argument usually comes from two camps: One being people who don't understand what AI actually is, its limitations, or the process of research commercialisation described in Q7. The other is fearmongering started by large commercial investors in AI who want to prohibit competition with their models which were first to the market. It is widely agreed in AI safety that attempting to supress the development of AI is one of the most dangerous options possible, as it will instead be developed in secret by organisations powerful enough to do so (like oppressive governments); while this may slow development, the end result will be much more dangerous and disruptive as it will be in the hands of a small number of powerful actors, will only be aligned to their interests, and there would be no gradual period of adjustment/adoption. A better approach would be to place greater emphasis on AI safety research and require adherence to ethical guidelines for developing and applying AI, just as we do with other sensitive research areas like medicine.